Bringing clarity when hiring a Data Science professional
Interviewing candidates for a Data Science position can be a challenge. I've developed hiring processes in the past and continue to work with some of my founder friends to tweak and refine their Data Science hiring initiatives.
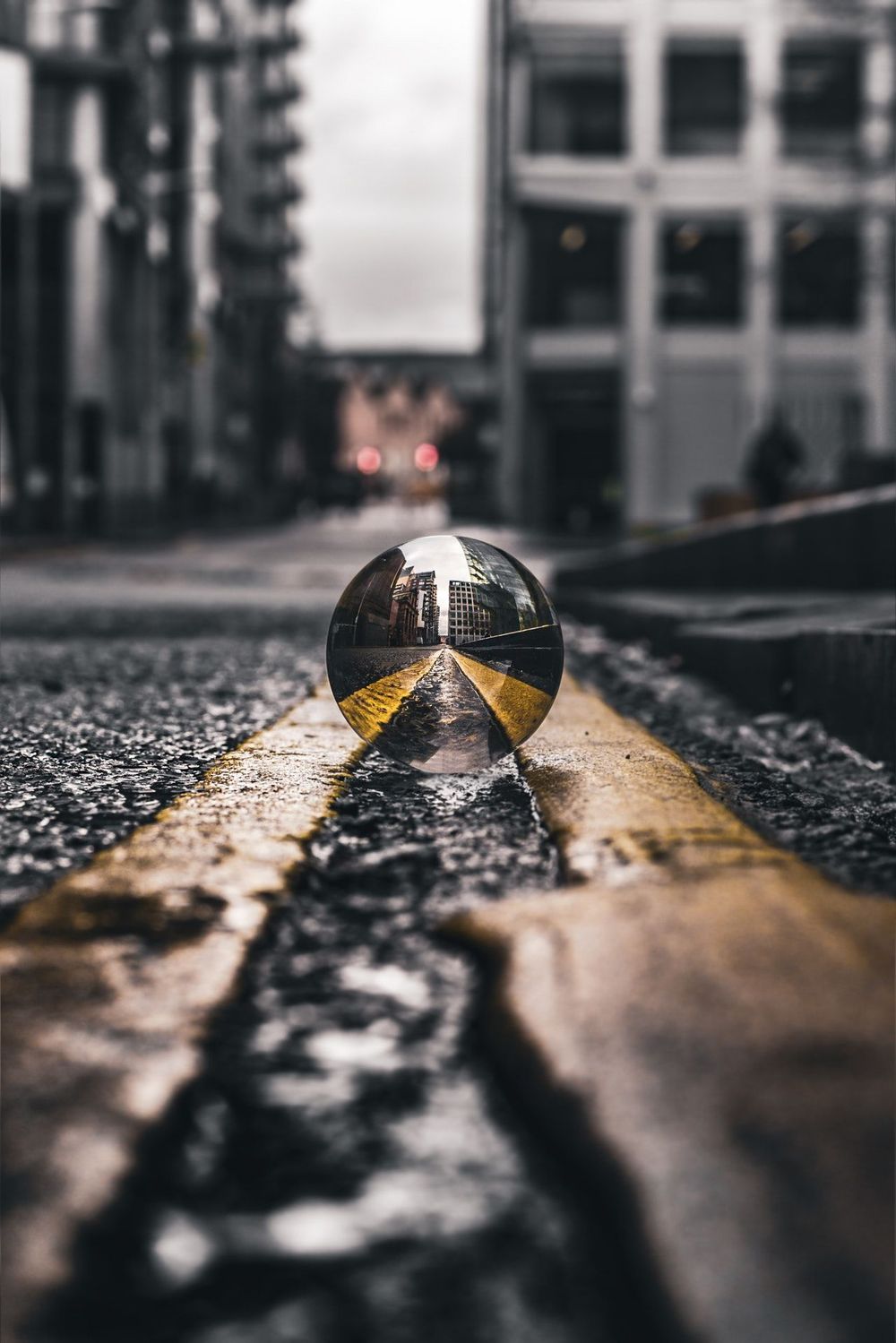
Interviewing candidates for a Data Science position can be a challenge. I've developed hiring processes in the past and continue to work with some of my founder friends to tweak and refine their Data Science hiring initiatives. Before I make some recommendations, I'd like to share some thoughts on the concerns I have with current hiring processes.
If a hiring process isn't yielding the desired results (i.e., impactful employees) the solution might just be to have a series of conversations with the hiring manager to understand more about the following;
- What is the burning problem that needs to be solved by the Data Scientist?
- When they join, what will their two weeks look like? Who will they be expected to speak with to gain context on their work expectations?
- Do you believe you have the infrastructure setup to give your Data Scientist the runway they need to be effective soon after they join?
- What are some tasks you expect them to accomplish on a day to day basis?
- What should they have accomplished on day 90? What should they have accomplished on day 180?
Where I'm going with this is to point out that hiring Data Science professionals is an endeavor that is fraught with uncertainty. And one reliable way to dispel doubt and bring in more clarity is to engage in a series of conversations regarding the DS mandate and the expected skills you are seeking in the DS hire.
I like to ask the hiring manager/founder to provide specific details along the following broad areas when hiring a Data Science professional.
- Create a specific project plan with context and expected deliverables for the first project they want their DS hire to address. More importantly, identify in as much detail as possible what will success look like once the project has been delivered. Kudos if you can identify more than 1 project you'd like addressed by your DS hire.
- Provide guidance on the necessary skills that is expected from the individual who is expected to work on the project. Are they expected to label data? Work with multiple stakeholders to clarify the initial problem statement? Do they need to develop dashboards before they work on developing models? Are they expected to build and deploy ML models? Any indications on which language choices have been made and libraries expected to be used? Are they expected to influence your dev team to log data necessary for downstream analysis? Will they be assigned a management champion when they make cross-functional requests?
- What if your DS gets stuck? Do you have access to DS leaders who can provide mentorship? Will you be willing to engage an expert freelancer to come in temporarily and provide support/guidance?
- What is the cadence of updates you expect from your DS? Will you be satisfied if you receive the same update three weeks in a row? If not, how do you plan to engage your DS so you can learn more about progress?
- Can you assign a monetary value to the impact you expect your DS to have? This is a tricky one and requires thinking deeply about KPIs and metrics that you need to have in place to measure new initiatives and interventions. But this conversation needs to be had as a way to force a deeper reflection on DS impact.
Finally, I will conclude this post by saying that as a DS professional myself, I thrive when I have a clear understanding of what problem needs to be solved. I also enjoy the process of discovering what the actual problem really is that needs to be addressed rather than the problem that is being held out as the one that needs a DS solution.
I believe that DS is both art and science. While this sounds trite, I'd like to use this to explain more about what I mean here. The art is all those "principles", "working models", "patterns" and "design" that one picks up as they work their way through developing and solving DS projects. Learning more of the art does come with experience but it also comes with reading broadly and cross-pollinating ideas from other domains.
The science is the tools and technologies that one chooses so they can give themselves the most leverage in their work. I like to gravitate towards tools, technologies and techniques that get me to do more with less effort and allow me to spend a lot more time thinking about the different problems that I can solve with the art and science of DS.