A World of Opportunities: Top Kaggle Competitions with Cash Prize
Learn about the standard competition categories in Kaggle which will help you apply all your Data Science knowledge while also giving you a chance to win cash prizes.
Kaggle competitions are there to help you earn by competing and you can show it off in your Resume too.
So, let's learn and earn!
Introduction
Kaggle is not just a platform for data science competitions but also they have a huge inventory of datasets. There are many notebooks related to various problems. There are short courses on many data science topics. They have discussion forums, research competitions, and much more. Kaggle has now become a platform for data scientists, so huge that it can be overwhelming to understand.
There are many competitions on Kaggle. The competitions are classified into different categories. Here are some of the competition categories :
1. Getting started competitions
These are the most accessible competition. These are the best competition for people just getting started with Kaggle. The competitions in this category do not have any prize money. The advantage of these competitions is that the solutions help to learn about interesting techniques and approaches.
1. Titanic- Machine learning for disaster (HOST- Kaggle):
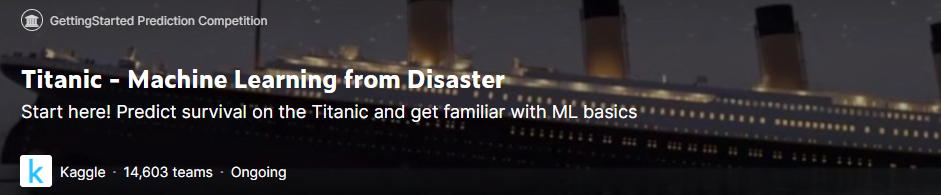
Dataset: Titanic dataset
This is the legendary Titanic ML competition – the best, first challenge for you to dive into ML competitions and familiarize yourself with how the Kaggle platform works. The competition is simple: use machine learning to create a model that predicts which passengers survived the Titanic shipwreck. In this, you are supposed to build a predictive model that answers the question: “what sorts of people were more likely to survive?” using passenger data (i.e. name, age, gender, socio-economic class, etc.).
2. House Prices using Advanced Regression Techniques(HOST-Kaggle):
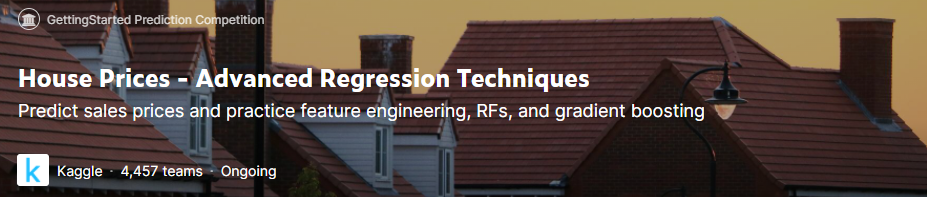
Dataset: Ames Housing dataset compiled by Dean De Cock
Predict sales prices and practice feature engineering, Random Forests, and gradient boosting. With 79 explanatory variables describing (almost) every aspect of residential homes in Ames, Iowa, this competition challenges you to predict the final price of each home. It uses advanced regression and classification algorithms such as decision trees, Random Forests, and other boosting techniques to classify the price of a house.
3. Digit Recognizer (Kaggle):
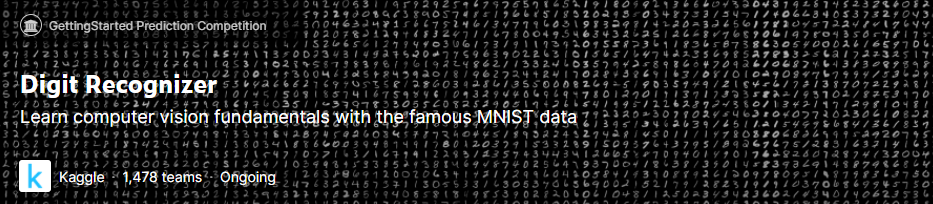
Dataset: MNIST data
Using this dataset your goal is to correctly identify digits from a dataset of tens of thousands of handwritten images. We’ve curated a set of tutorial-style kernels which cover everything from regression to neural networks. We encourage you to experiment with different algorithms to learn first-hand what works well and how techniques compare. It requires SVM and K-nearest neighbors classification techniques and also simple neural networks.
2. Featured Competitions
The featured competitions are the most sort after. These competitions generally attract huge prize money. They also attract top talents from all over the world. It is the best place to learn from the experts. The discussion forums of these competitions are goldmines. They hold so much information that can be very helpful. A prerequisite to entering a featured competition is having a strong knowledge of the basics.
1. G- Research Crypto Forecasting:
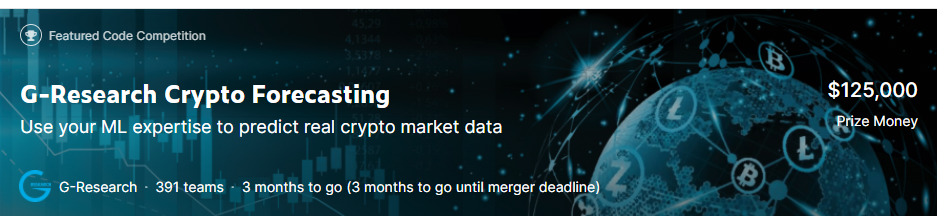
- About: G-Research is Europe's leading quantitative finance research firm. They club their expertise with machine learning, big data, and some of the most advanced technology available to predict movements in financial markets. They are the biggest questions solver in finance.
- The Objective of the Competition: In this competition, you'll use your machine learning expertise to forecast short-term returns in 14 popular cryptocurrencies. We have amassed a dataset of millions of rows of high-frequency market data dating back to 2018 which you can use to build your model. Since 2018, interest in the crypto market has exploded, so the volatility and correlation structure in the data is likely to be highly non-stationary. The successful contestant will pay careful attention to these considerations, and in the process gain valuable insight into the art and science of financial forecasting.
2. Merck Molecular Activity Challenge:

- About: Merck (known as MSD outside the U.S. and Canada) has been inventing for life, bringing forward medicines and vaccines for many of the world’s most challenging diseases in pursuit of their mission to save and improve lives. They demonstrate commitment to patients and population health by increasing access to health care through far-reaching policies, programs and partnerships. They are researching to prevent and treat diseases that threaten people and animals – including cancer, infectious diseases, such as HIV and Ebola, and emerging animal diseases.
- When developing new medicines it is important to identify molecules that are highly active toward their intended targets but not toward other targets that might cause side effects. The challenge is based on 15 molecular activity data sets, each for a biologically relevant target. Each row corresponds to a molecule and contains descriptors derived from that molecule's chemical structure.
- The Objective of the Competition: The objective of this competition is to identify the best statistical techniques for predicting biological activities of different molecules, both on- and off-target, given numerical descriptors generated from their chemical structures. In addition to the prediction competition, Merck is also hosting a visualization challenge with a $2,000 prize for the most insightful and elegant graphical representations of the data.
3. Chaii-Hindi and Tamil Question Answering:
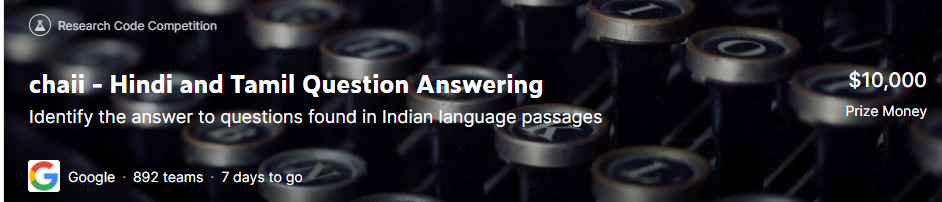
- About: Chaii (Challenge in AI for India) is a Google Research India initiative created for sparking AI applications to address some of the pressing problems in India and to find unique ways to address them. Starting with a focus on NLU, chaii hopes to make progress towards multilingual modeling, as language diversity is significantly underserved on the web.
- The Objective of the Competition: In this competition, your goal is to predict answers to real questions about Wikipedia articles. You will use chaii-1, a new question-answering dataset with question-answer pairs. The dataset covers Hindi and Tamil, collected without the use of translation. It provides a realistic information-seeking task with questions written by native-speaking expert data annotators. You will be provided with a baseline model and inference code to build upon. If successful, you can improve upon the baseline performance of NLU models in Indian languages. The results could improve the web experience for many of the nearly 1.4 billion people of India. Additionally, you’ll contribute to multilingual NLP, which could be applied beyond the languages in this competition.
4. The National Football League - Sports Analytics:
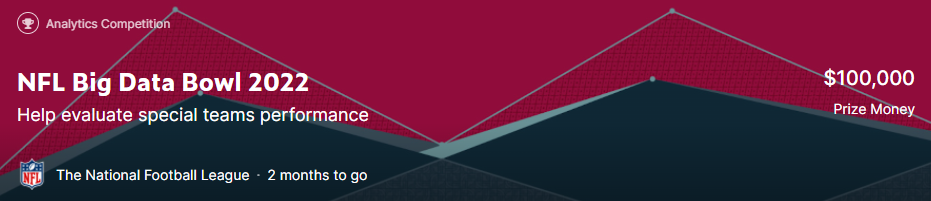
- About: The NFL is America's most popular sports league. Founded in 1920, the organization behind American football has developed the model for the successful modern sports league. They're committed to advancing every aspect of the game, including the lesser researched special teams. In this competition, you’ll quantify what happens in special teams' plays. You might create a new special teams metric, quantify team or individual strategies, rank players, or even something we haven’t considered. If successful, your effort may even be adopted by the NFL for on-air distribution, and you can watch future games knowing you had a hand in improving America's most popular sports league.
- The Objective of the Competition: This model created by you would help evaluate special teams performance and eventually would help players improve their performance. The NFL's Next Gen Stats (NGS) tracking data from all 2018-2020 special teams plays provides location information for each special teams player, wherever they are on the field, and includes their speed, acceleration, and direction. Also, for the first time in Big Data Bowl history, participants can utilize scouting data from PFF, which supplements the tracking data with football-specific metrics that coaches find critical to team success.
5. Prudential Life Insurance Assessment:
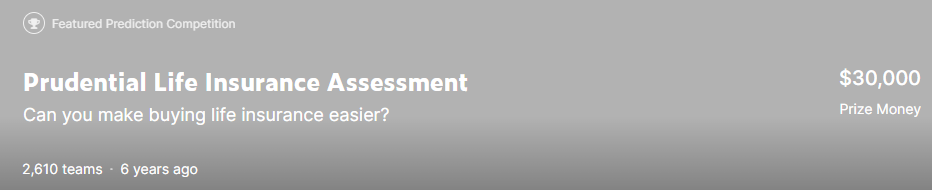
- About: Prudential Financial, Inc. is an American Fortune Global 500 and Fortune 500 company whose subsidiaries provide insurance, investment management, and other financial products and services to both retail and institutional customers throughout the United States and in over 40 other countries.
- In a one-click shopping world with on-demand everything, the life insurance application process is antiquated. Customers provide extensive information to identify risk classification and eligibility, including scheduling medical exams, a process that takes an average of 30 days. The result? People don't want to associate with it. That’s why only 40% of U.S. households own individual life insurance. Prudential wants to make it quicker and less labour-intensive for new and existing customers to get a quote while maintaining privacy boundaries.
- The Objective of the Competition: Develop a predictive model that accurately classifies risk using a more automated approach. The results will help Prudential better understand the predictive power of the data points in the existing assessment, enabling us to significantly streamline the process.
6. Quora Question Pairs:
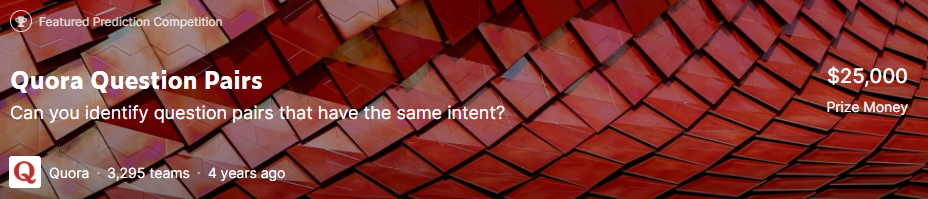
- About: Quora is a place to gain and share knowledge—about anything. It’s a platform to ask questions and connect with people who contribute unique insights and quality answers. This empowers people to learn from each other and to better understand the world.
- Over 100 million people visit Quora every month, so it's no surprise that many people ask similarly worded questions. Multiple questions with the same intent can cause seekers to spend more time finding the best answer to their question and make writers feel they need to answer multiple versions of the same question. Quora values canonical questions because they provide a better experience to active seekers and writers, and offer more value to both of these groups in the long term.
- The Objective of the Competition: Quora uses a Random Forest model to identify duplicate questions. In this competition, Kagglers are challenged to tackle this natural language processing problem by applying advanced techniques to classify whether question pairs are duplicates or not. Doing so will make it easier to find high-quality answers to questions resulting in an improved experience for Quora writers, seekers, and readers.
7. Home Credit Default Risk:
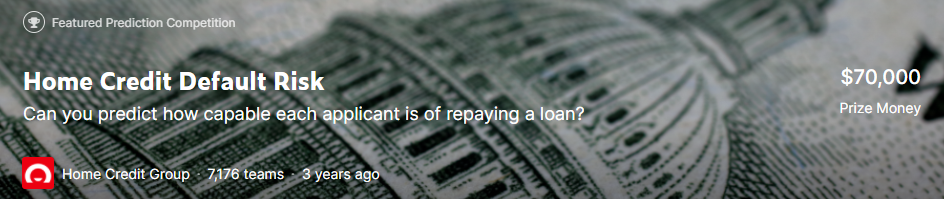
- About: Home Credit is an international non-bank financial institution founded in 1997 in the Czech Republic and headquartered in the Netherlands. The company operates in 9 countries and focuses on instalment lending primarily to people with little or no credit history, point-of-sale (POS) loans, cash loans, and revolving loan products through their online and physical distribution network. Customers typically start with point-of-sale financing in stores. Reliable customers can then adopt broader consumer credit products and ultimately we progress to providing fully-fledged branch-based consumer lending.
- Home Credit strives to broaden financial inclusion for the unbanked population by providing a positive and safe borrowing experience. In order to make sure this underserved population has a positive loan experience, Home Credit makes use of a variety of alternative data--including telco and transactional information--to predict their clients' repayment abilities.
- The Objective of the Competition: While Home Credit is currently using various statistical and machine learning methods to make these predictions, they're challenging Kagglers to help them unlock the full potential of their data. Doing so will ensure that clients capable of repayment are not rejected and that loans are given with a principal, maturity, and repayment calendar that will empower their clients to be successful.
8. Mercari Price Suggestion Challenge:
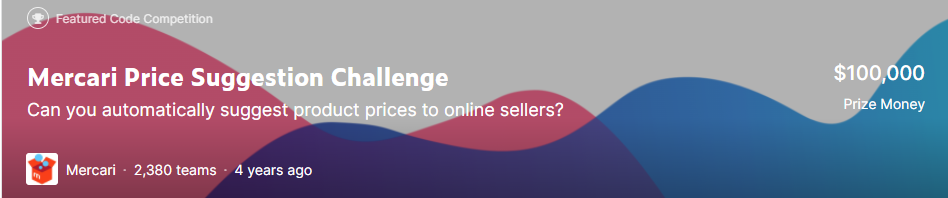
- About: Mercari is Japan’s biggest community-powered shopping app, knows this problem deeply. They’d like to offer pricing suggestions to sellers, but this is tough because their sellers are enabled to put just about anything, or any bundle of things, on Mercari's marketplace.
- The Objective of the Competition: Mercari’s challenge is to build an algorithm that automatically suggests the right product prices as product pricing gets even harder at scale, considering just how many products are sold online. Clothing has strong seasonal pricing trends and is heavily influenced by brand names, while electronics have fluctuating prices based on product specs. You’ll be provided user-inputted text descriptions of their products, including details like product category name, brand name, and item condition.
9. Cervical Cancer Screening:
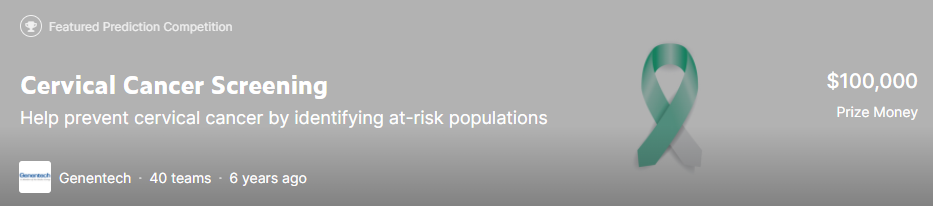
- About: Genentech is a leading biotechnology company that discovers, develops, manufactures and commercializes medicines to treat patients with serious or life-threatening medical conditions. The company, a member of the Roche Group, has headquarters in South San Francisco, California.
- The Objective of the Competition: Cervical cancer is the third most common cancer in women worldwide, affecting over 500,000 women and resulting in approximately 275,000 deaths every year. After reading these statistics, you may be surprised to hear that cervical cancer is potentially preventable and curable.
- Genentech, through this competition, is asking you to join their mission to help prevent cervical cancer. Given a dataset of de-identified health records, the challenge is to predict which women will not be screened for cervical cancer on the recommended schedule. Identifying at-risk populations will make education and other intervention efforts more effective, ideally ultimately reducing the number of women who die from this disease.
10. dunnhumby & hack/reduce Product Launch Challenge:

- About: dunnhumby empowers brands and retailers to do this through more relevant and engaging experiences. They help their clients build Customer-First strategies with cutting-edge solutions, and never stop innovating. dunnhumby empowers brands & retailers to do this through more relevant and engaging experiences.
- The Objective of the Competition: This competition asks you to predict how successful each of the numbers of product launches will be 26 weeks after the launch, based only on information up to the 13th week after the launch.
- Prediction accuracy will be evaluated based on the root mean square logarithmic error:

Where,
i is a product.
n is the total number of products.
p is the predicted sales volume (units sold in week 26).
a is the actual sales volume (units sold in week 26).
Note: the metric is calculated using the natural log.
Conclusion
These are some of the standard competition categories in Kaggle. Most of these competitions are single stage. It means that the datasets and the required information are all provided and the team with the best accuracy wins the competition.
There are some competitions which are two-stages, Research Competition. In these 2-staged competitions, the participants are first evaluated based on the initial dataset. Those who successfully complete the first stage moves to Stage 2. The team with the best score in Stage 2 is the winner.
We will look into the research competition in the upcoming articles and will study together how can you can win these competitions and earn money while also learning a lot even during competing.
It was all for now, until next time!