Unlocking Opportunities: Dive into the World of Data Annotation and Labeling Jobs
Explore the world of data annotation opportunities! Unleash the power of AI by labeling and curating data. Join the journey where every annotation shapes the future of machine learning!
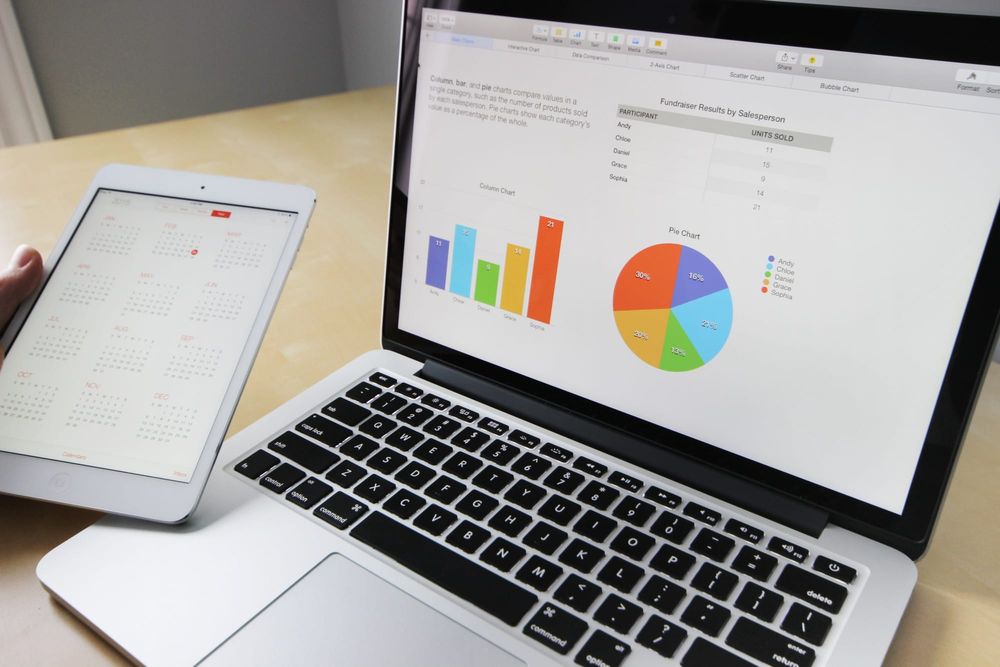
In the ever-evolving landscape of machine learning and data science, the value and the importance of data has reached unparalleled heights. Amongst the vast sea of data-related concepts, data annotation and labeling have emerged as crucial processes!
But firstly, let us find out what these terms mean!
Data annotation and labeling
Data annotation is a broader term where it refers to a group of metadata addition techniques, which when carried out add context into the datasets. Labeling is a specific subset of annotation that includes the meticulous assignment of labels or categories to individual data points which lead to the development of more accurate and efficient machine learning models.
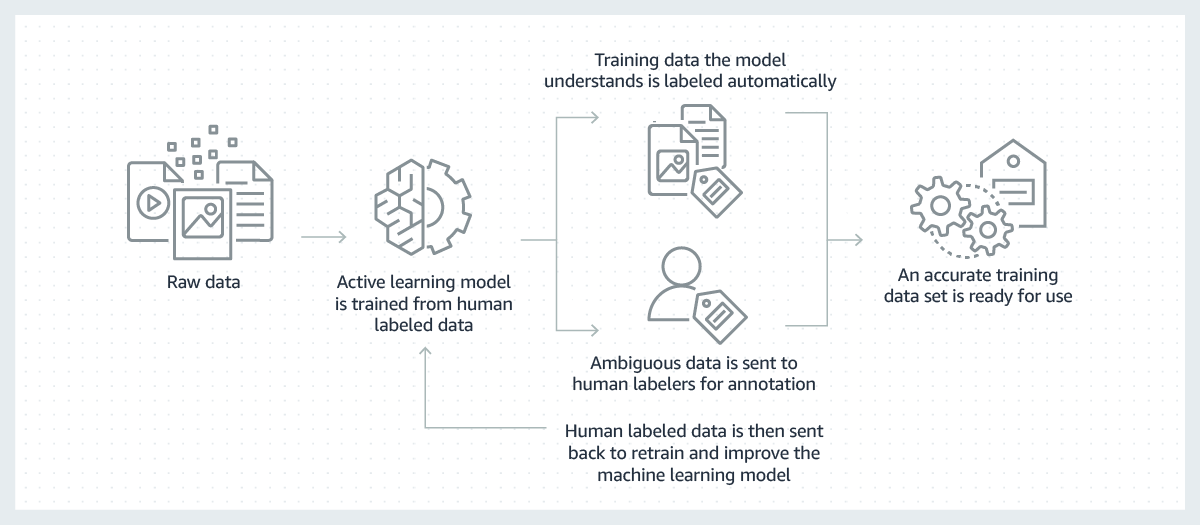
These can include both humans and machines working together harmoniously, where humans can get fully involved in labeling the data completely or a machine learning model for labeling data can be built and trained to speed up the creation of training datasets.
For more details, you can refer to this article.
Types of data annotation

Data annotation can be classified into:
- Image annotation: Involves adding metadata to images which includes labeling with bounding boxes(to define the spatial limits of objects within an image), object classes(to specify the type of object contained within these boxes), and segmentation (involves outlining object contours with pixel-level accuracy, offering precise details about the shape and structure of objects) to provide essential context. Mostly applied in object detection, image recognition, and autonomous vehicles.
- Audio annotation: Involves the addition of labels to audio files, including phonemes, phonetic transcriptions, and speaker identification. Widely applied in speech recognition and natural language processing, this is instrumental in training machine learning models to comprehend speech patterns and recognize spoken words. Phonetic annotation labels individual sounds or phonemes, while speaker identification involves recognizing different speakers in an audio recording. In applications like call centers, speaker identification aids in discerning speakers during conversations.
- Video annotation: Involves the addition of labels to videos for object detection, action recognition, and activity recognition. This is crucial for machines to comprehend visual data and make informed decisions. Widely applied in security and surveillance, it helps identify and track objects or individuals in video feeds, contributing to tasks like recognizing car details, detecting faces, and monitoring movement. The insights gained from video annotation are valuable for enhancing public safety, identifying security threats, and analyzing traffic patterns.
- Text annotation: Focuses on adding labels to text data. It encompasses identifying and labeling elements like named entities, sentiment, and part-of-speech in the text. Named entities include specific entities like people or locations, sentiment analysis gauges the tone of the text and part-of-speech tagging identifies grammatical components. Applied extensively in natural language processing and text classification, text annotation is crucial for extracting valuable information from textual data and facilitating effective analysis.
- Semantic annotation: Enriches data, such as text or multimedia content, with metadata to convey meaning and context, surpassing simple labeling. This process captures semantic relationships, entities, and concepts within the data, enhancing machine interpretability. In natural language processing (NLP), it involves tagging words or sentences with specific meanings for tasks like named entity recognition and sentiment analysis. In multimedia, semantic annotation identifies objects, actions or scenes.
Tools for data annotation
Amazon SageMaker Ground Truth((provided by Amazon Web Services(AWS)) is a powerful platform that offers comprehensive data labeling capabilities across various data types, such as text, images, video, audio, and point cloud. This tool allows users to label data effectively, ensuring the creation of high-quality training datasets for a wide range of machine learning use cases.
Whether you're annotating textual information, images, video sequences, audio recordings, or three-dimensional point cloud data, it provides the tools and flexibility needed to train machine learning models with precision and efficiency.
Also, it offers enhanced features like automated data labeling and annotation consolidation. The platform supports various workflows, including built-in task types and custom labeling workflows, making it a valuable resource for machine learning practitioners. Moreover, it introduces the concept of a "human-in-the-loop," allowing human annotators to work alongside automated processes, further refining the quality of labeled datasets.
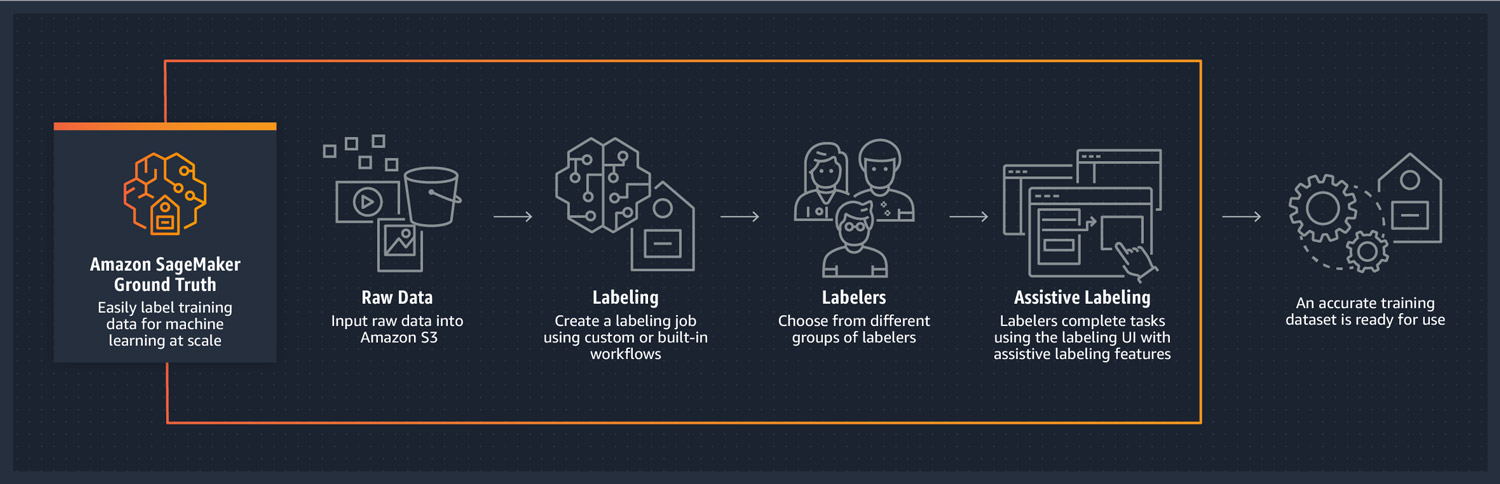
SuperAnnotate is a very popular data annotation platform, serving as a crucial element in the data labeling process. Boasting a cutting-edge annotation tool accommodating various data types, including images, video, text, LiDAR, and audio, it prioritizes fast and high-quality annotation. The platform enables efficient collaboration with stakeholders, ensuring annotation accuracy and process streamlining. It offers specialized features for diverse machine learning tasks, such as LLM annotation, image and video annotation, text annotation, audio annotation, and LiDAR annotation, catering to different industry needs.
It extends its impact across industries like agriculture, healthcare, insurance, sports, robotics, autonomous driving, aerial imagery, NLP, and security and surveillance, addressing a broad spectrum of use cases. Beyond its powerful annotation tool, the platform provides annotation services through a global marketplace, efficient project and quality management, and AI data management for precise dataset creation.
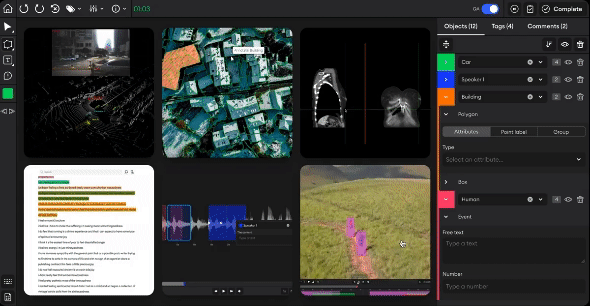
Label Studio emerges as a versatile open-source data labeling platform, offering flexibility for fine-tuning LLMs, preparing training data, and validating AI models across various data types. The platform covers a wide range of applications, including computer vision, audio and speech, NLP, documents, chatbots, transcripts, robots, sensors, IoT devices, and video. For computer vision tasks, it supports image classification, object detection, and semantic segmentation, while in audio and speech applications, it facilitates classification, speaker diarization, emotion recognition, and audio transcription. NLP capabilities include document classification, named entity extraction, question answering, and sentiment analysis.
Additionally, Label Studio caters to tasks related to robots, sensors, IoT devices, and video, providing functionalities such as time series classification, segmentation, event recognition, video classification, object tracking, and assisted labeling.
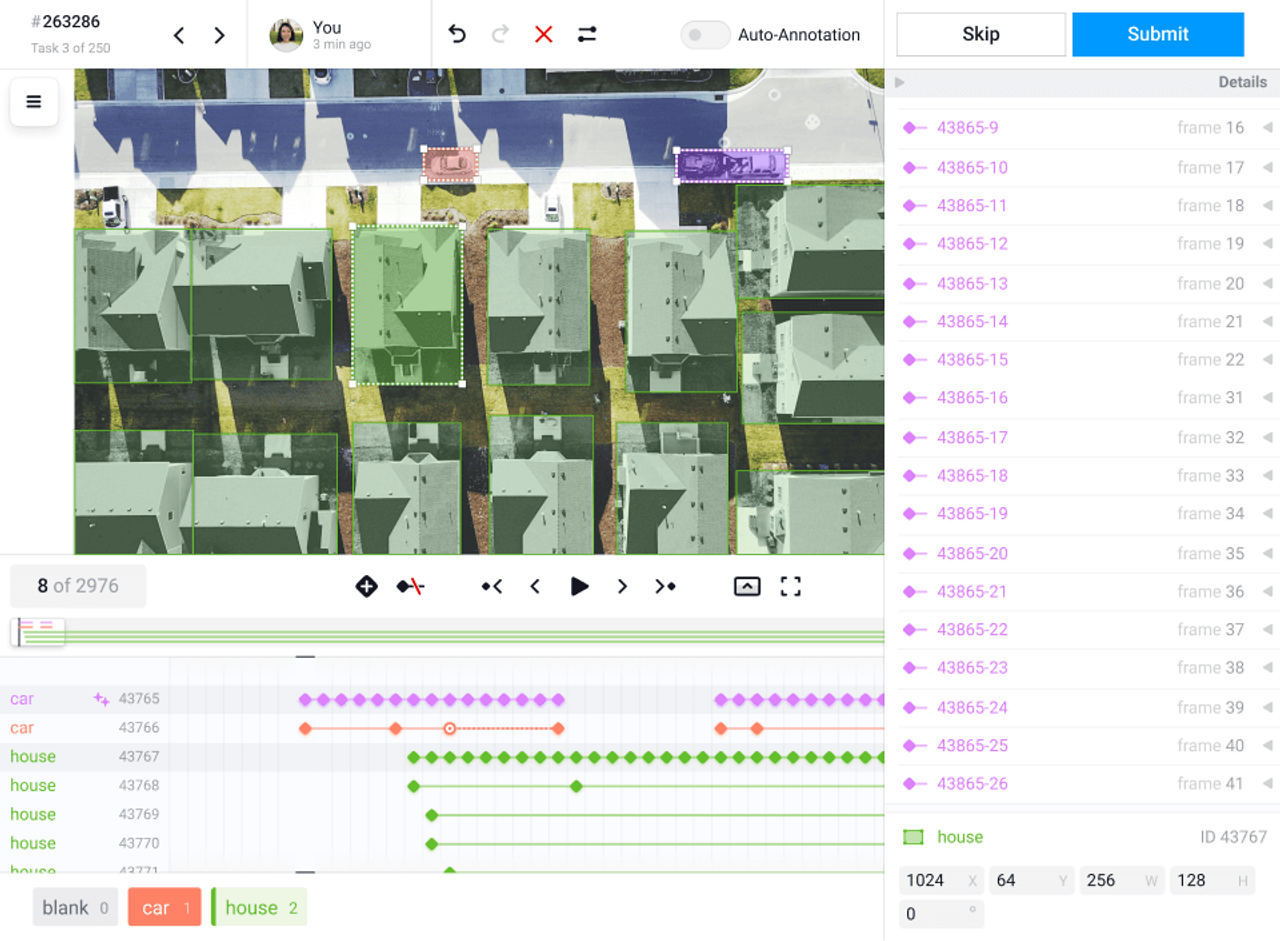
Scale AI is a tool which seamlessly integrates AI-driven techniques with human-in-the-loop processes, ensuring unparalleled efficiency and scalability in delivering high-quality labeled datasets. The platform's proficiency spans diverse data types, from 3D and images to mapping, text, and audio, making it a comprehensive solution for data labeling that significantly enhances the performance of Language Model Models (LLMs) and generative models.
At the core of Scale AI's offers tools covering data curation, dataset management, testing, model evaluation, and model comparison. This toolkit empowers users to intelligently manage datasets, identify high-value data for labeling, and optimize labeling budgets effectively. In essence, Scale AI provides a cutting-edge platform that combines advanced AI technologies with human expertise, with RLHF serving as a game-changing methodology.
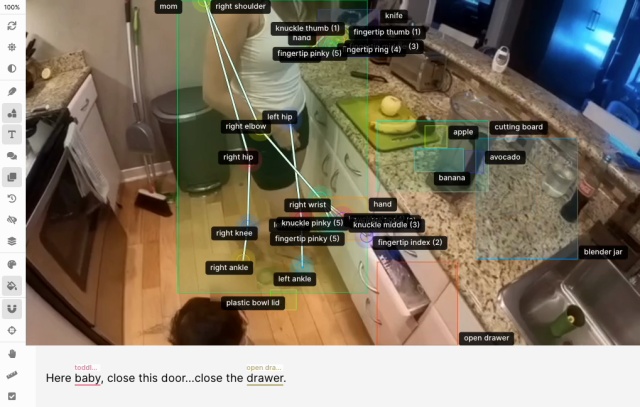
Market Size and Growth Trends in Data Collection and Labeling
The global data collection and labeling market, valued at USD 2.22 billion in 2022, are expected to exhibit substantial growth with a projected compound annual growth rate (CAGR) of 28.9% from 2023 to 2030. This market is set to experience increased adoption due to its diverse applications, including extracting business insights from shared images and enhancing safety features in autonomous vehicles.
Key Trends and Insights:
- Market size Insights: The global market is expected to reach USD 17.10 billion by 2030, with North America dominating the market in 2022, accounting for over 35% of global revenue.
In regards to the Asia Pacific region, the following image represents the market:
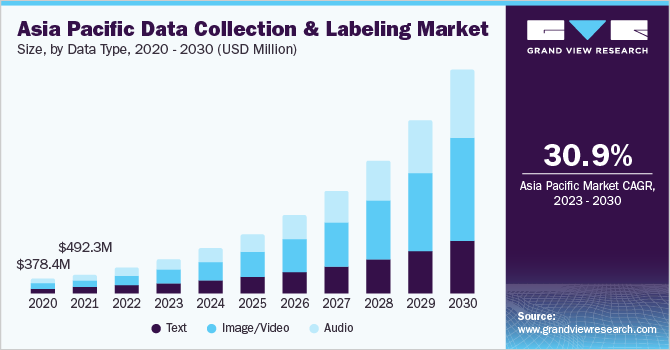
- Market Growth Drivers:
- Increased use of technology to organize collections of untagged photos and derive business insights from images.
- Contributions to autonomous vehicle safety aspects, such as wear detection, terrain detection, emergency vehicle detection, and condition monitoring.
- Machine learning integration across a multitude of industries, including robotics and drone applications, automatic picture arrangement on visual websites, and facial recognition on social networking sites.
- Rising popularity of social media monitoring for digital marketing growth and safety and security applications, increasing significance of decisions supported by data, resulting in an ongoing flow of data for analysis and insights.
- Industry applications:
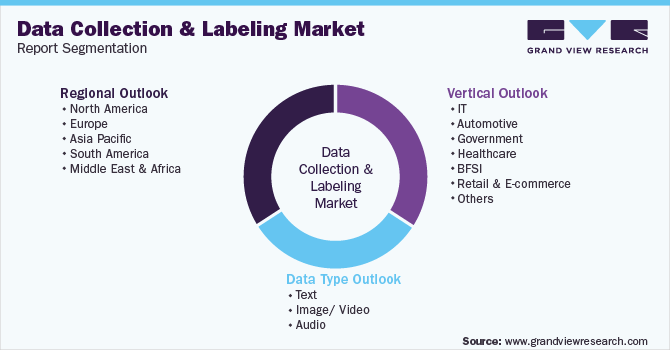
- Data Type Insights: With over 36% of the worldwide revenue share in 2022, image/video data type led the market, fueled by the growing application of computer vision across a range of sectors. In 2022, text data type constituted a noteworthy portion, owing to its utilization in e-commerce and clinical research.
- Vertical insights: Due to the extensive deployment of AI, the IT industry held over 30% of the market for data collecting and labeling in 2022. The expected expansion in healthcare will depend on AI applications, which means that proper data labeling is required. Retail and e-commerce users can look for products by using photographs taken with their smartphones and image labeling. By improving obstacle detection and traffic signal reading, the automobile industry benefits from the adoption of data annotation in autonomous vehicles.
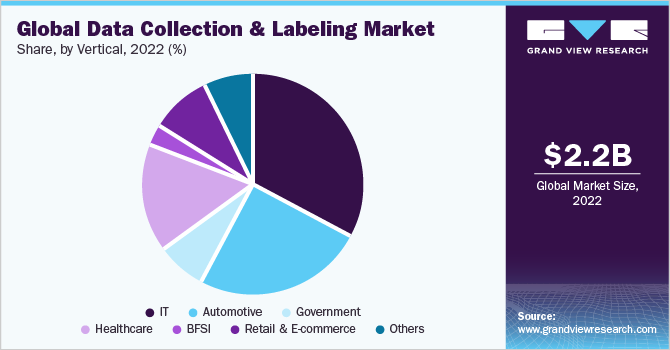
- Regional insights: With over 35% of the global revenue share in 2022, North America led the data collection and labeling market. This was due to the growing popularity of cloud-based media services, as well as the incorporation of mobile computing platforms, artificial intelligence, and digital commerce. The development of car obstacle detection technologies is expected to propel the European market's significant growth, while the Asia Pacific region is predicted to grow at the fastest rate because of the region's widespread use of mobile devices, data processing capabilities, and popularity of social networking sites—particularly in emerging economies like China and India. The Asia Pacific region's growing need for data gathering and annotation is partly fueled by face recognition apps and real-name registration rules.
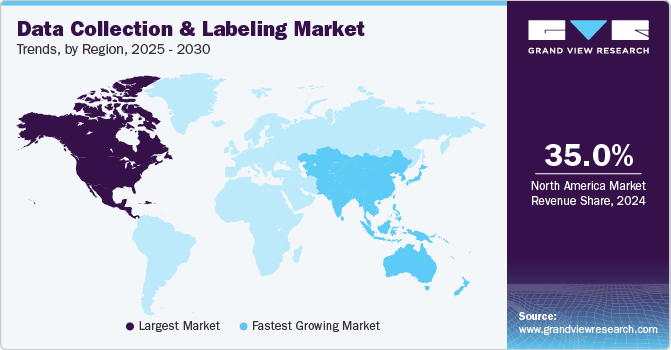
- Key Companies and Market Share:
- Market players are focusing on increasing their customer base through strategic initiatives, including collaborations, acquisitions & mergers and partnerships.
- Notable companies in the market include Reality AI, Summa Linguae Technologies, Alegion, Labelbox, Scale AI, Appen and more.
Future Trends and Challenges: The market anticipates heightened demand in healthcare, particularly in medical imaging, leveraging data collection and annotation to train AI systems for disease detection. Simultaneously, advancements in sentiment analysis and social media monitoring propel the application of text labeling. To address challenges like inaccuracy in data annotation, the industry is actively deploying automated technologies, marking a significant step toward enhancing precision and efficiency in data labeling processes.
Top skills needed to secure a job as data annotator/ labeler
It's a combination of specific skills that are essential for effective objectives to be satisfied. These skills can be put into two broad categories, mainly hard and soft skills.
Essential hard skills
SQL(Structured Query Language) proficiency is one of the most vital hard skill needed in the industry today, it allows us for accessing and manipulating databases which contain vast amounts of data required in the field of machine learning.Knowledge and clarity of programming languages such as Python, Java or R is another factor for annotators when developing tools or scripts for automating repetitive annotation tasks. These help in formulating algorithms for faster and more consistent processing.Another necessary skill is the requirement of attention to detail, precision in the terms of annotation accuracy plays a big role for high quality training set production of data.Finally, based on the particular type of annotation required, the knowledge and experience in working with specific annotation tools would be required.
Essential soft skills
- In the initial aspect, the capability to effectively manage time and prioritize tasks is crucial for meeting project deadlines. Since the annotation process can be time-intensive, understanding the duration of each task and arranging them in order of importance is essential for ensuring timely project completion.
- Additionally, possessing strong critical thinking skills is indispensable when dealing with intricate data sets. Data annotators must be adept at making well-informed decisions regarding the relevance of specific annotations to achieve precise and accurate outcomes.
- Moreover, soft skills that can be applied across various contexts, such as communication and collaboration, are pivotal. Data analysts collaborate with diverse teams within their organization and effective communication and teamwork are essential for maximizing the potential of data. Maintaining cohesion among team members is facilitated by a combination of teamwork and adaptability when working on projects.
- Also, essential skills for excelling in this role include strong problem-solving abilities to analyze complex issues and identify optimal solutions. Solid numerical skills, including a grasp of statistical concepts and meticulous attention to detail, are also vital to ensure accuracy when working with data. Furthermore, expertise in data visualization is crucial for creating compelling visual representations that enhance understanding and facilitate communication with stakeholders across different departments.
Companies hiring for data annotation roles
Here are a few companies, each with its unique focus and roles:
AI Startups
Appen: As a global leader in human-annotated data, Appen provides a variety of data annotation services essential for enhancing machine learning models. Roles within Appen include Data Annotator and AI/ML Data Annotation Analyst. The company specializes in text, audio, and image annotation, offering opportunities for remote work in annotation projects.

Surge AI: One of the largest RLHF(Reinforcement learning from Human Feedback) platforms based in the US, Surge AI specializes in Natural Language Processing (NLP) and advanced labeling tasks. The company focuses on delivering high-quality data to top tech companies and researchers, addressing the challenges of NLP and other advanced labeling tasks with an elite workforce and modern APIs. Surge AI actively engages human data annotators to ensure quality in their labeling tasks.
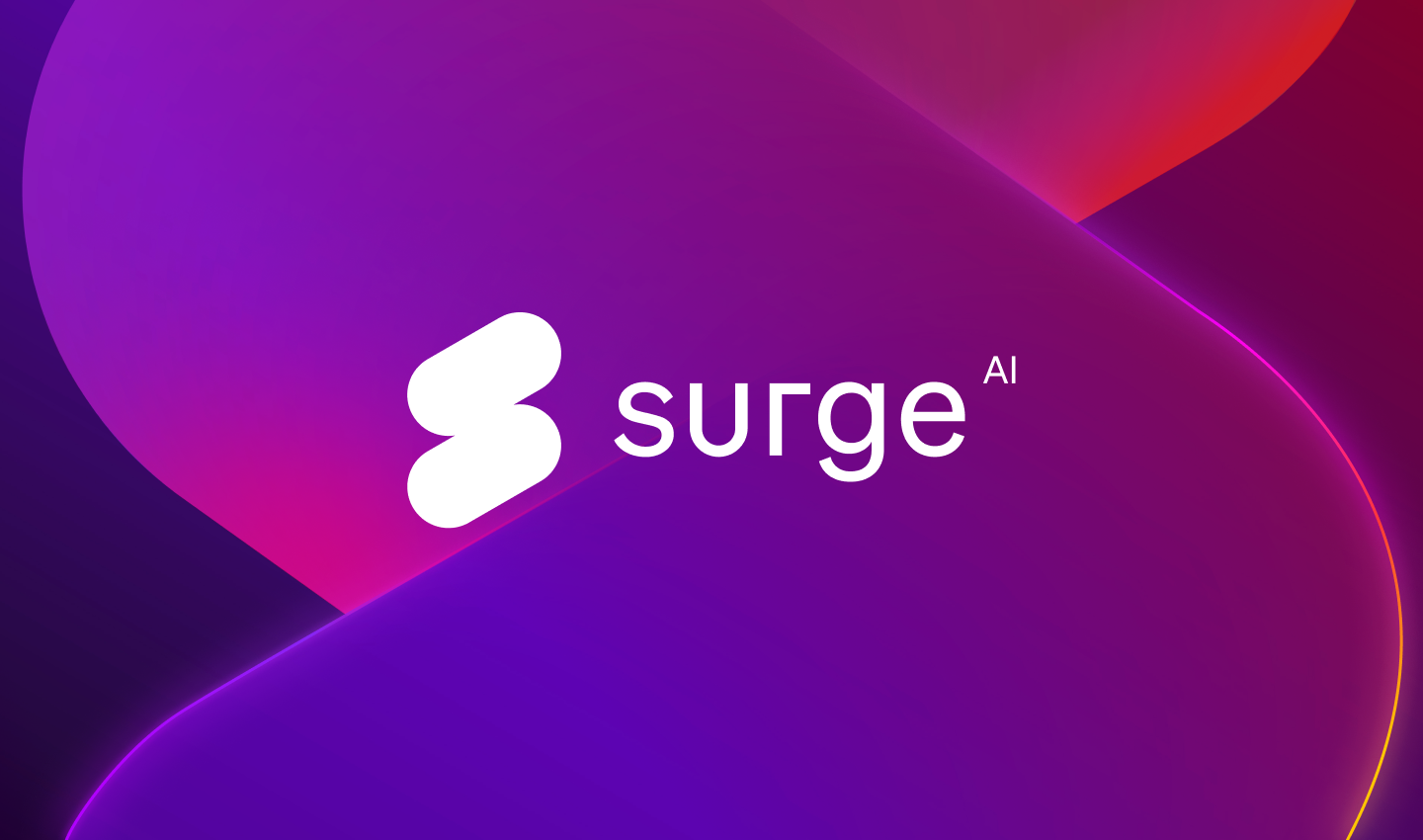
Scale AI: A prominent provider of data annotation services, Scale AI is known for creating meticulously labeled datasets for diverse machine learning applications. Common roles at Scale AI include Data Labeler or Annotation Specialist, involving tasks such as image segmentation and classification. The company supports remote work, allowing individuals to contribute to annotation projects from different locations.
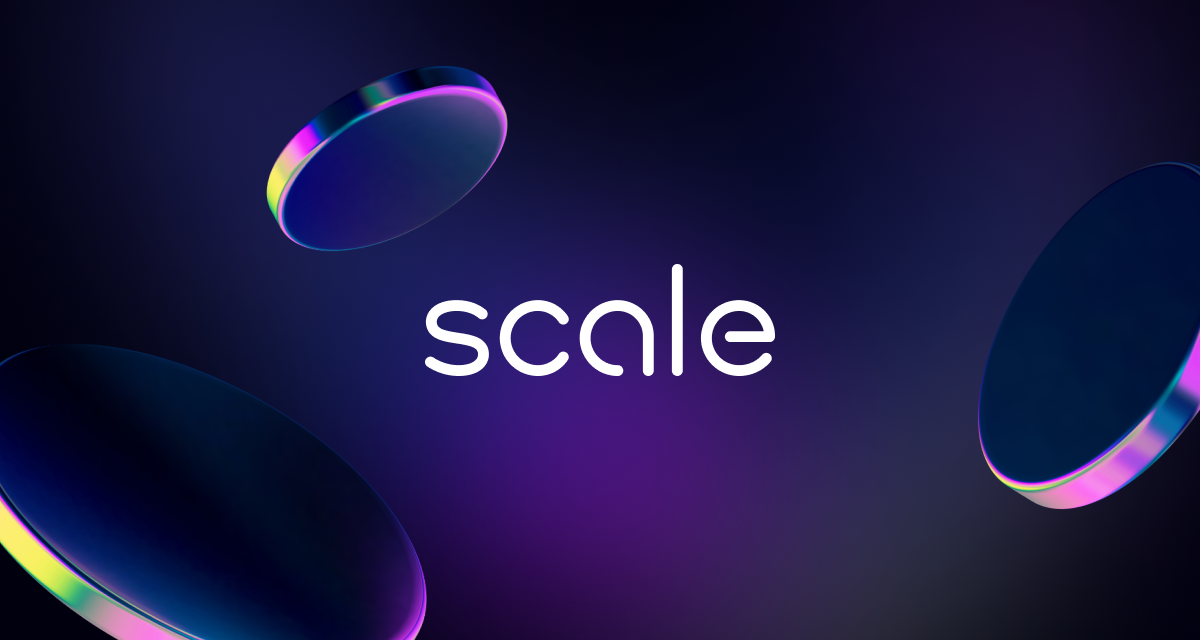
Level AI: A startup innovating in the Voice AI space, it focuses on revolutionizing the customer sales experience. Roles at Level AI, such as Data Annotators, involve classifying and labeling English text and audio files. These individuals ensure annotation quality and provide constructive feedback.

Other AI startups
Sizzle: Sizzle is an exciting startup in the gaming world with AI-driven automation of gaming highlights. In the role of a Data Labeler at Sizzle, individuals gather training data from gaming videos on platforms like Twitch and YouTube. This includes labeling and annotating video data by rendering 3D models, working closely with AI engineers to improve model performance.Expertia AI: Expertia AI specializes in HR Tech, offering products like Virtual Recruiter and Expertia Career Site. The role of Textual Data Annotator involves annotating and labeling data to support machine learning algorithms. The position requires expertise in textual data annotation, data cleaning, and segmentation.Snorkel AI: Snorkel AI provides an AI platform that enables programmatic data labeling, efficient model training, and rapid application deployment. The platform allows users to label data at scale, fine-tune language models, and build specialist models 100x faster, reducing the manual effort involved in data annotation. Snorkel AI welcomes human data annotators as part of their workflow.V7 Labs: Based in the United Kingdom, V7 Labs offers an AI platform that accelerates model release and reduces errors in various fields. The company's data engine automates the labeling process, employing programmatic labeling workflows that use AI models and minimal human steering to apply labels to data at scale, these include human data labelers as part of their solution.
Fintech companies
Yubi (formerly CredAvenue): Yubi is a fintech company redefining global debt markets through AI. In the role of a Data Labeler at Yubi, individuals contribute to annotating financial data, crucial for training machine learning models. Yubi's platforms, including Yubi Loans and Yubi Invest, leverage data annotation to provide innovative financial solutions.JPMorgan Chase: The machine learning team at JPMorgan Chase combines cutting-edge machine learning techniques with the company's unique data assets to optimize various financial processes. Roles, such as AI/ML Data Annotation Analyst, involve annotating and labeling image, video, text, and audio data via computer using internal software programs. The role contributes to training machine learning models in the finance domain.
Where can one apply for these roles?
One can apply through various methods, some of these are:
Firstly, you can explore by going through the Careers page of the particular organization you are interested to do data annotation for. Based on your interests, you can easily filter out and apply( you can take referrals from people that are already a part of the organization), the recruiters will reach out to you looking at the past experience as well as your skills.Searching through Linkedin jobs, where you can look for a role based on the keywords entered, this feature too provides multiple filtering options such as experience level, remote/onsite, companies that you are specifically looking for. Here is an example:
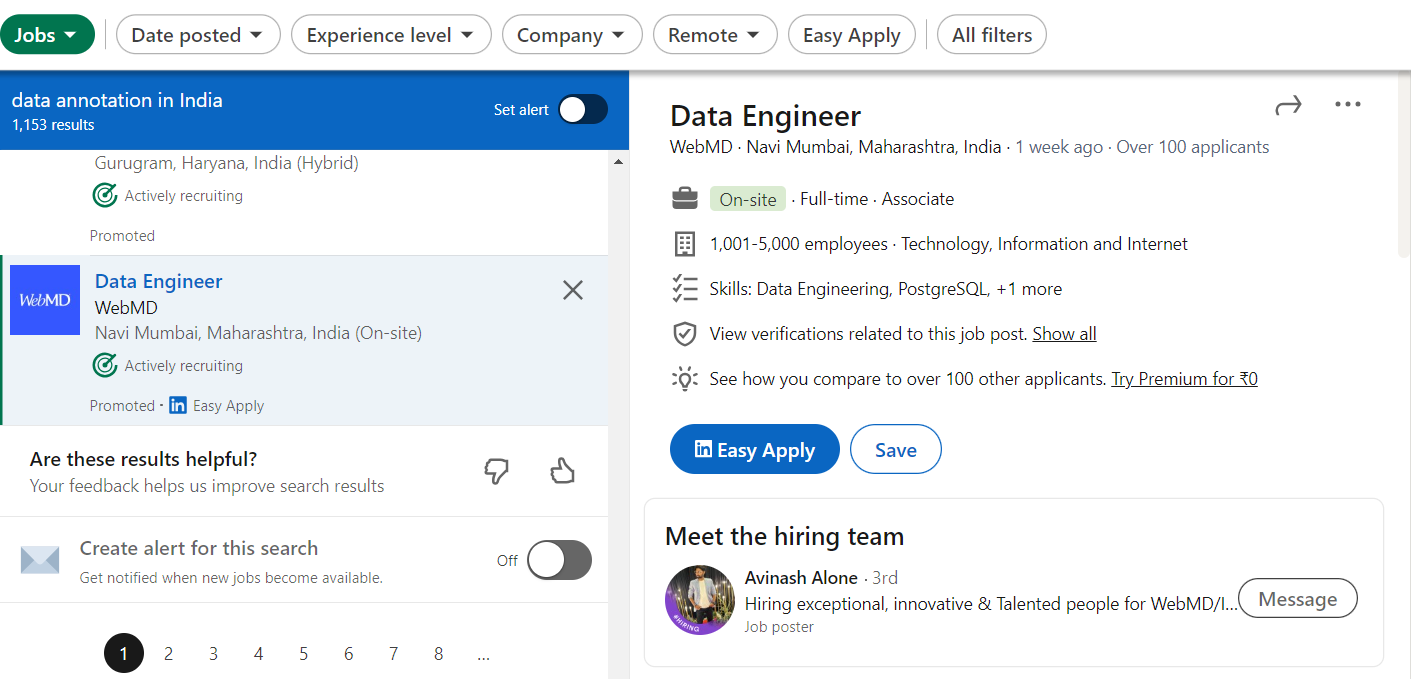
Other alternative websites such as Indeed, Naukri, Glassdoor are also available, these are updated regularly so do keep an eye out on a timely basis.
You can also check out freelancing platforms like Upwork or Freelancer where companies and individuals often post short-term data annotation projects. Having a profile showcasing your skills and experience combined with actively applying for these projects can help build a good profile. The image below shows an example:
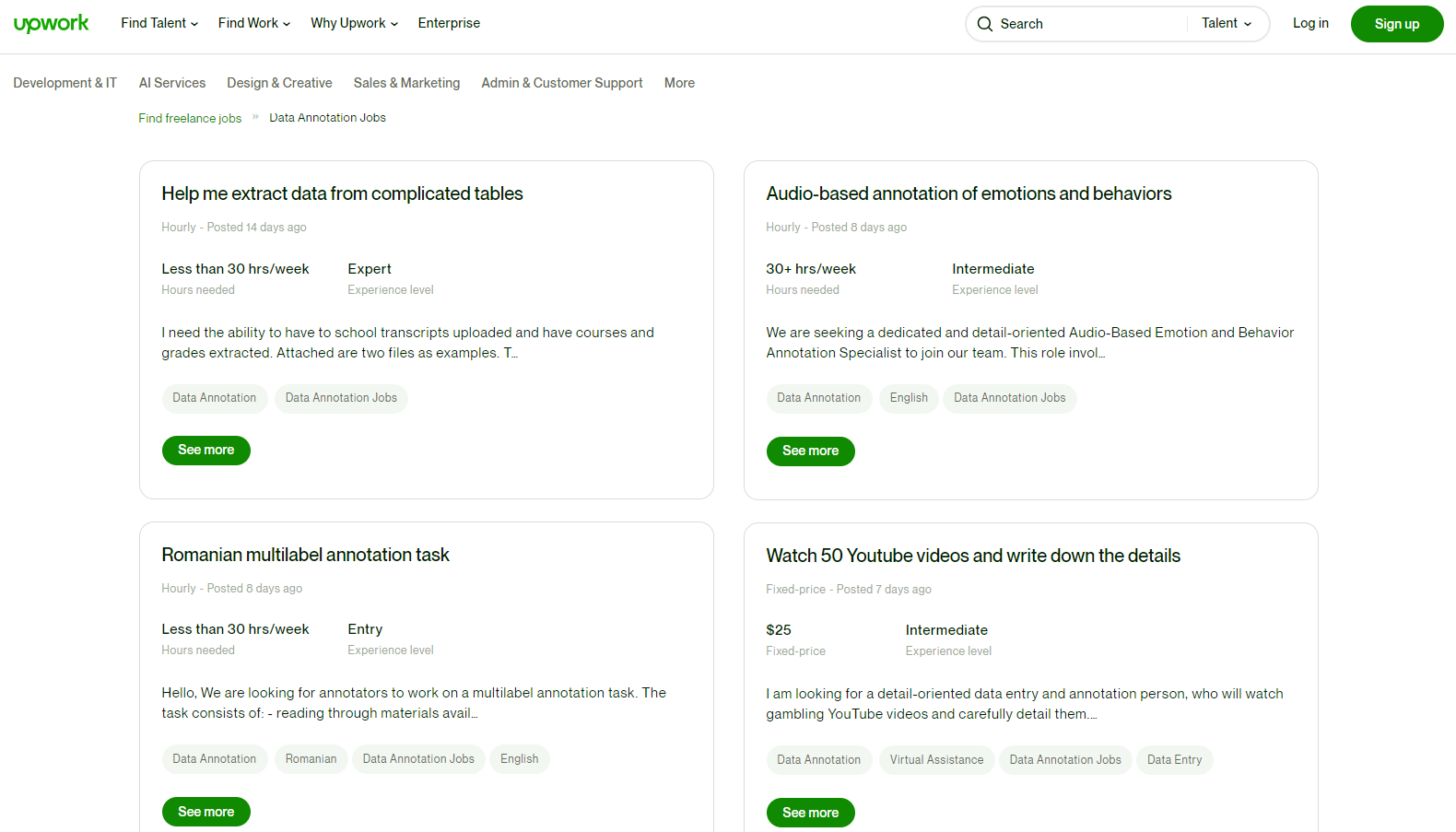
Networking with professionals in the AI and machine learning industry, either online or at industry events can really play a crucial role. Attending meetups, webinars, or conferences where you can connect with professionals and potentially discover job opportunities. Websites such as Eventbrite and Meetup help in discovering events happening online or at your location.
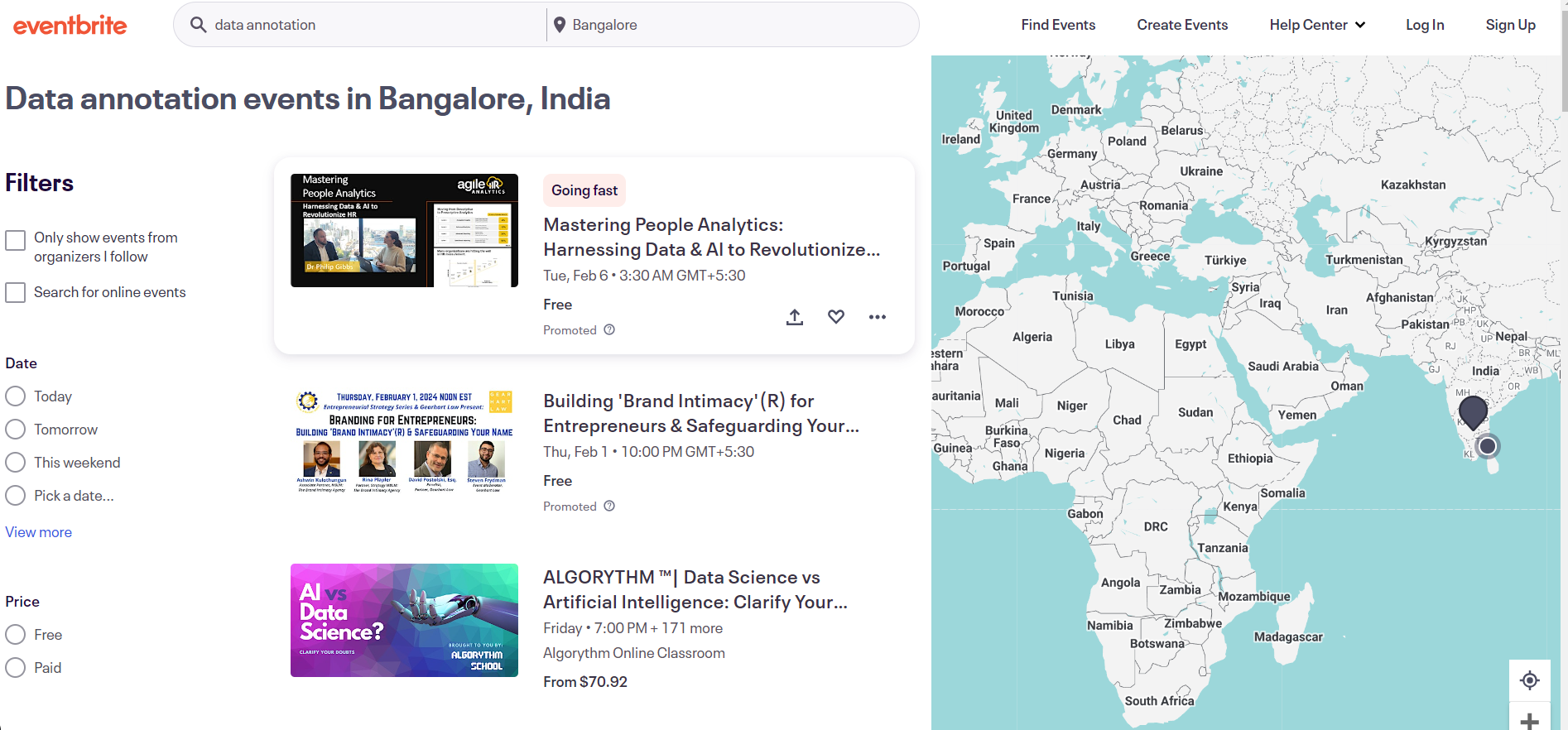
Online Training and Certifications related to data annotation & machine learning enhances your qualifications (do mention them on your resume to stand out to potential employers). A few of them include this course from Skillshare, another course is from DTouch.
Summary
There are vast opportunities available in the field of data annotation and labeling for people looking for meaningful work in the technology sector. For the advancement of cutting-edge technologies like artificial intelligence and machine learning, accurate data annotation is essential.
A career in data annotation offers both professional and personal growth, along with the opportunity to contribute to technological advancements. This industry has a strong feeling of community, which offers opportunity for networking and insightful information.
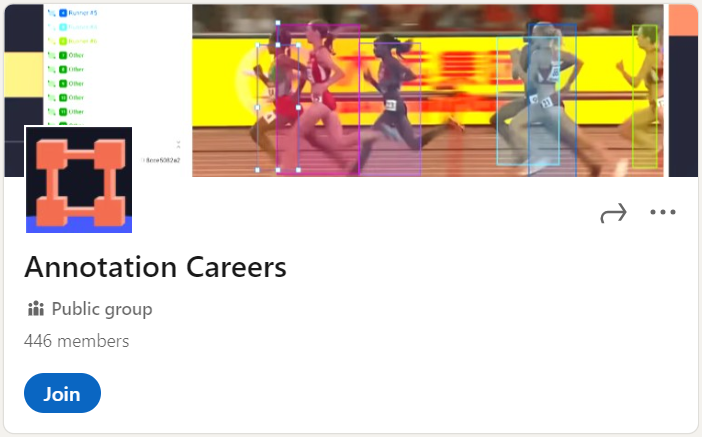
Opportunities for career progression are abundant as the volume of data increases and so does the demand for qualified data annotators. Take advantage of the opportunities, network with industry experts, and establish yourself as a leader in the rapidly changing field of data annotation.
Your adventure into this fascinating field is waiting for you; seize the chance to advance your career!